‘I need evidence yesterday’: Gesund raises $2 million to provide algorithm-validating data
It’s one thing to develop a medical algorithm, quite another to prove that it actually works. To do that, you need one crucial thing that’s hard to come by: medical data. And one startup is ready to provide that in spades, along with the tools to make validation studies easier. Gesund, founded in 2021, emerged […]
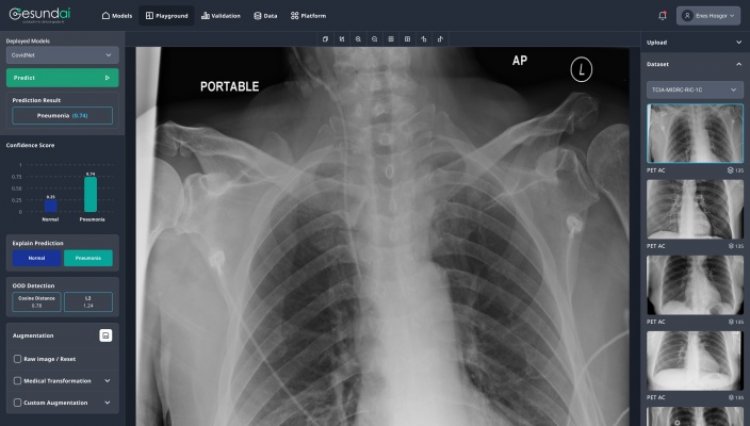
It’s one thing to develop a medical algorithm, quite another to prove that it actually works. To do that, you need one crucial thing that’s hard to come by: medical data. And one startup is ready to provide that in spades, along with the tools to make validation studies easier.
Gesund, founded in 2021, emerged from stealth this week with a $2 million seed round led by 500 Global. The company has already come a long way, boasting viable platforms, 30 clients in their sales pipeline, and revenue expected this quarter, CEO and founder Enes Hosgor told TechCrunch.
Gesund is basically a Contract Research Organization (CRO) for AI companies developing medical algorithms, or or academics testing their own models. The same way a CRO might design a clinical trial for a drug or medical device company, Gesund’s platform curates data that allows AI companies to test their own products and creates the IT infrastructure to make that comparison run smoothly.
“I like to think of us as a machine learning ops company,” said Hosgor. “We don’t do algorithms.”
A medical algorithm is only as good as the data it’s trained on, and there is evidence that getting diverse and usable datasets can be a challenge. For example, a study published in JAMA in 2020 analyzed 74 scientific papers describing deep learning algorithms across disciplines like radiology, ophthalmology, dermatology, pathology, gastroenterology and pathology. 71 percent of data used in these studies came from New York, California and Massachusetts.
34 US states did not contribute any data to the pipeline that had been used to train these algorithms, calling into question how generalizable they might be to a wider population.
The issue also exists across different types of healthcare providers. You could train an algorithm on data collected at a large, esteemed, academic hospital. But if you want to deploy that in a small community hospital there’s no guarantee it will work in that very different setting.
Taken together, the datasets used to train algorithms are, in general, smaller than they should be, according to one meta-review of 152 studies published in the BMJ. Naturally, there are some algorithmic success stories, but this is an industry wide problem.
Technology alone can’t solve all these issues; You can’t sort or provide data that isn’t there in the first place. Think genetic studies for people of non-European ancestry, which are sorely lacking. But Gesund is focused narrowly on an issue where tech might help: making existing data easier to access and creating partnerships that open up new avenues for data sharing.
Gesund’s data pipeline comes from “existing data sharing agreements in place with clinical sites,” said Hosegor. Right now, Gesund is focused on imaging data collected at the University of Chicago Medical Center, Massachusetts General Hospital, and Berlin’s Charité. (The company plans to extend beyond radiology in the future).
Aggregating and delivering data for use in machine learning applications is also being done by others, like the Nightingale Open Science Project, which will freely provide clinical datasets to researchers (not affiliated with Google’s controversial “Project Nightingale”). But while the data itself is a critical piece of this, it’s really the technology stack that Hosegor sees as the company’s secret weapon.
“Everybody does ML on the cloud,” explained Hosegor. “And because your average healthcare provider doesn’t have a cloud, all that goes out the window,” he said. “We have built this technology stack that can reside on premises, inside a hospital firewall. It does not rely on any third party managed services, which are the bread and butter of machine learning.”
From there, the platform includes a “low code” interface. In short, physicians and providers can basically drag and drop the datasets they need and test their own algorithms against that data.
“We’re about six months old, but we hit the ground running and we built this first product that allows model owners to run their algorithms against data to produce accuracy metrics on the fly, in high compliance environments where they don’t have access to cloud resources. That’s our secret sauce,” he explained.
At the moment, Gesund, somewhat like Nightingale, is providing some of its services for free. The company’s Community Edition allows academics with existing algorithms to test their algorithms for free (but they’ll have to upload their own datasets).
Meanwhile it’s the AI companies that will foot the bill for the company’s “premium” version. This, says Hosegor, will give the paying customers access to proprietary datasets. And there’s evidence they’ll pay for the data they need. At the moment, Gesund claims to have a pipeline of 30 potential clients, and expects to generate revenue this quarter.
“We were at RSNA in Chicago last November and every single AI company we talked to said ‘yes, I need evidence yesterday.’”
The $2 million pre-seed round represents all of Gesund’s funding, but Hosegor expects the company to raise again this year. In the near future the company will focus on R&D and expanding its clinical partnerships in the US and Europe.