Synthesis AI raises $17M to generate synthetic data for computer vision
Synthesis AI, a startup developing a platform that generates synthetic data to train AI systems, today announced that it raised $17 million in a Series A funding round led by 468 Capital with participation from Sorenson Ventures and Strawberry Creek Ventures, Bee Partners, PJC, iRobot Ventures, Boom Capital and Kubera Venture Capital. CEO and cofounder […]
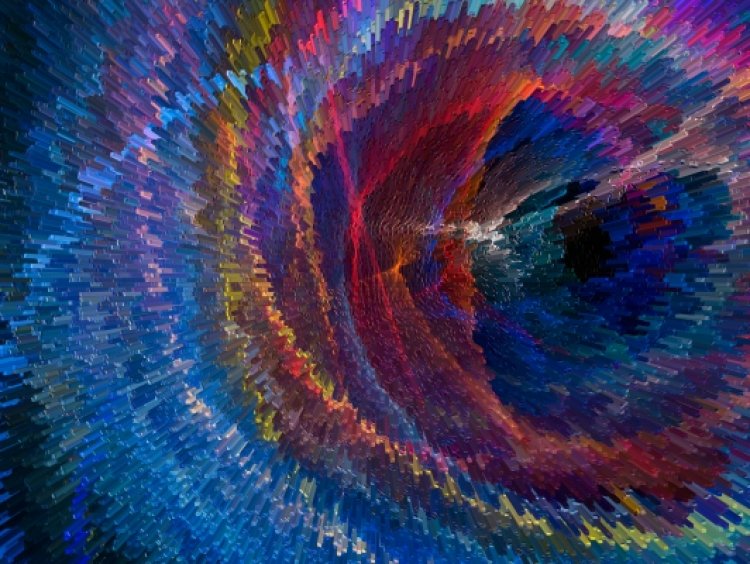
Synthesis AI, a startup developing a platform that generates synthetic data to train AI systems, today announced that it raised $17 million in a Series A funding round led by 468 Capital with participation from Sorenson Ventures and Strawberry Creek Ventures, Bee Partners, PJC, iRobot Ventures, Boom Capital and Kubera Venture Capital. CEO and cofounder Yashar Behzadi says that the proceeds will be put toward product R&D, growing the company’s team, and expanding research — particularly in the area of mixed real and synthetic data.
Synthetic data, or data that’s created artificially rather than captured from the real world, is coming into wider use in data science as the demand for AI systems grows. The benefits are obvious: While collecting real-world data to develop an AI system is costly and labor-intensive, a theoretically infinite amount of synthetic data can be generated to fit any criteria. For example, a developer could use synthetic images of cars and other vehicles to develop a system that can differentiate between makes and models.
Unsurprisingly, Gartner predicts that 60% of the data used for the development of AI and analytics projects will be synthetic by 2024. One survey called the use of synthetic data “one of the most promising general techniques on the rise in [AI].”
But synthetic data has limitations. While it can mimic many properties of real data, it isn’t an exact copy. And the quality of synthetic data is dependent on the quality of the algorithm that created it.
Behzadi, of course, asserts that Synthesis has taken meaningful steps toward overcoming these technical hurdles. A former scientist at IT government services firm SAIC and the creator of PopSlate, a smartphone case with a built-in E Ink display, Behzadi founded Synthesis in AI in 2019 with the goal of — in his words — “solving the data issue in AI and transform[ing] the computer vision paradigm.
“As companies develop new hardware, new models, or expand their geographic and customer base, new training data is required to ensure models perform adequately,” Behzadi told TechCrunch via email. “Companies are also struggling with ethical issues related to model bias and consumer privacy in human-centered products. It is clear that a new paradigm is required to build the next generation of computer vision.”
In most AI systems, labels — which can come in the form of captions or annotations — are used during the development process to “teach” the system to recognize certain objects. Teams normally have to painstakingly add labels to real-world images, but synthetic tools like Synthesis’ eliminate the need — in theory.
Synthesis’ cloud-based platform allows companies to generate synthetic image data with labels using a combination of AI, procedural generation, and VFX rendering technologies. For customers developing algorithms to tackle challenges like recognizing faces and monitoring drivers, for instance, Synthesis generated roughly 100,000 “synthetic people” spanning different genders, ages, BMIs, skin tones, and ethnicities. Through the platform, data scientists could customize the avatars’ poses as well as their hair, facial hair, apparel (e.g., masks and glasses), and environmental aspects like the lighting and even the “lens type” of the virtual camera.
“Leading companies in the AR, VR, and metaverse space are using our diverse digital humans and accompanying rich set of 3D facial and body landmarks to build more realistic and emotive avatars,” Behzadi said. “[Meanwhile,] our smartphone and consumer device customers are using synthetic data to understand the performance of various camera modules …. Several of our customers are building a car driver and occupant sensing system. They leveraged synthetic data of thousands of individuals in the car cabin across various situations and environments to determine the optimal camera placement and overall configuration to ensure the best performance.”
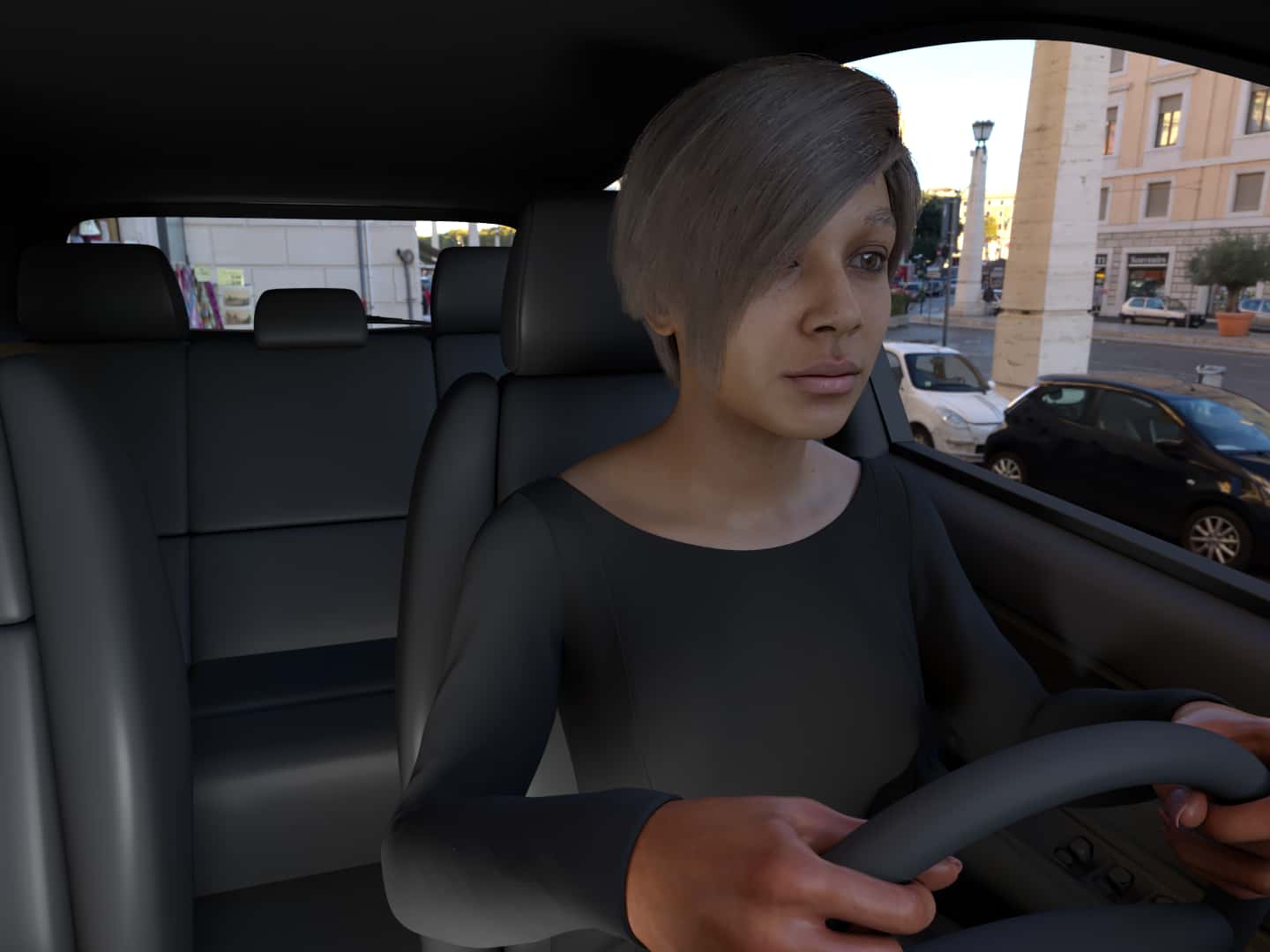
One of Synthesis AI’s digital avatars.
Some of the domains that Synthesis endorses are controversial, it’s worth pointing out — like facial recognition and “emotion sensing.” Gender and racial biases are a well–documented phenomenon in facial analysis, attributable to shortcomings in the datasets used to train the algorithms. (Generally speaking, an algorithm developing using images of people with homogenous facial structures and colors will perform worse on “face types” to which it hasn’t been exposed.) Recent research highlights the consequences, showing that some production systems classify emotions expressed by Black people as more negative. Computer vision-powered tools like Zoom’s virtual backgrounds and Twitter’s automatic photo cropping, too, have historically disfavored people with darker skin.
But Behzadi is of the optimistic belief that Synthesis can reduce these biases by generating examples of data — e.g., diverse faces — that’d otherwise go uncollected. He also claims that Synthesis’ synthetic data confers privacy and fair use advantages, mainly in that it’s not tied to personally identifiable information (although some research disagrees) and isn’t copyrighted (unlike many of the images on the public web).
“In addition to creating more capable models, Synthesis is focused on the ethical development of AI by reducing bias, preserving privacy, and democratizing access … [The platform] provides perfectly labeled data on-demand at orders of magnitude increased speed and reduced cost compared to human-in-the-loop labeling approaches,” Behzadi said. “AI is driven by high-quality labeled data. As the AI space shifts from model-centric to data-centric AI, data becomes the key competitive driving force.”
Indeed, synthetic data — depending on how it’s applied — has the potential to address many of the development challenges plaguing companies attempting to operationalize AI. Recently, MIT researchers found a way to classify images using synthetic data. Nvidia researchers have explored a way to use synthetic data created in virtual environments to train robots to pick up objects. And nearly every major autonomous vehicle company uses simulation data to supplement the real-world data they collect from cars on the road.
But again, not all synthetic data is created equal. Datasets need to be transformed in order to make them useable by the systems that create synthetic data, and assumptions made during the transformations can lead to undesirable results. A STAT report found that Watson Health, IBM’s beleaguered life sciences division, often gave poor and unsafe cancer treatment advice because the platform’s models were trained using erroneous, synthetic patient records rather than real data. And in a January 2020 study, researchers at Arizona State University showed that an AI system trained on a dataset of images of professors could create highly realistic synthetic faces — but synthetic faces that were mostly male and white, because it amplified biases contained in the original dataset.
Matthew Guzdial, an assistant computer science professor at the University of Alberta, points out that Synthesis’ own white paper acknowledges that training a model on synthetic data alone generally causes it to do a worse job.
“I don’t see anything that really stands out here [with Synthesis’ platform]. It’s pretty standard, synthetic-data-wise. In some cases they’re able to use synthetic data in combination with real data to help a model usefully generalize,” he told TechCrunch via email. “[G]enerally I steer my students away from using synthetic data as I find that it’s too easy to introduce bias that actually makes your end model worse … Since synthetic data is generated in some algorithmic fashion (e.g., with a function), the easiest thing for a model to learn is to just replicate the behaviour of that function, rather than the actual problem you’re trying to approximate.”
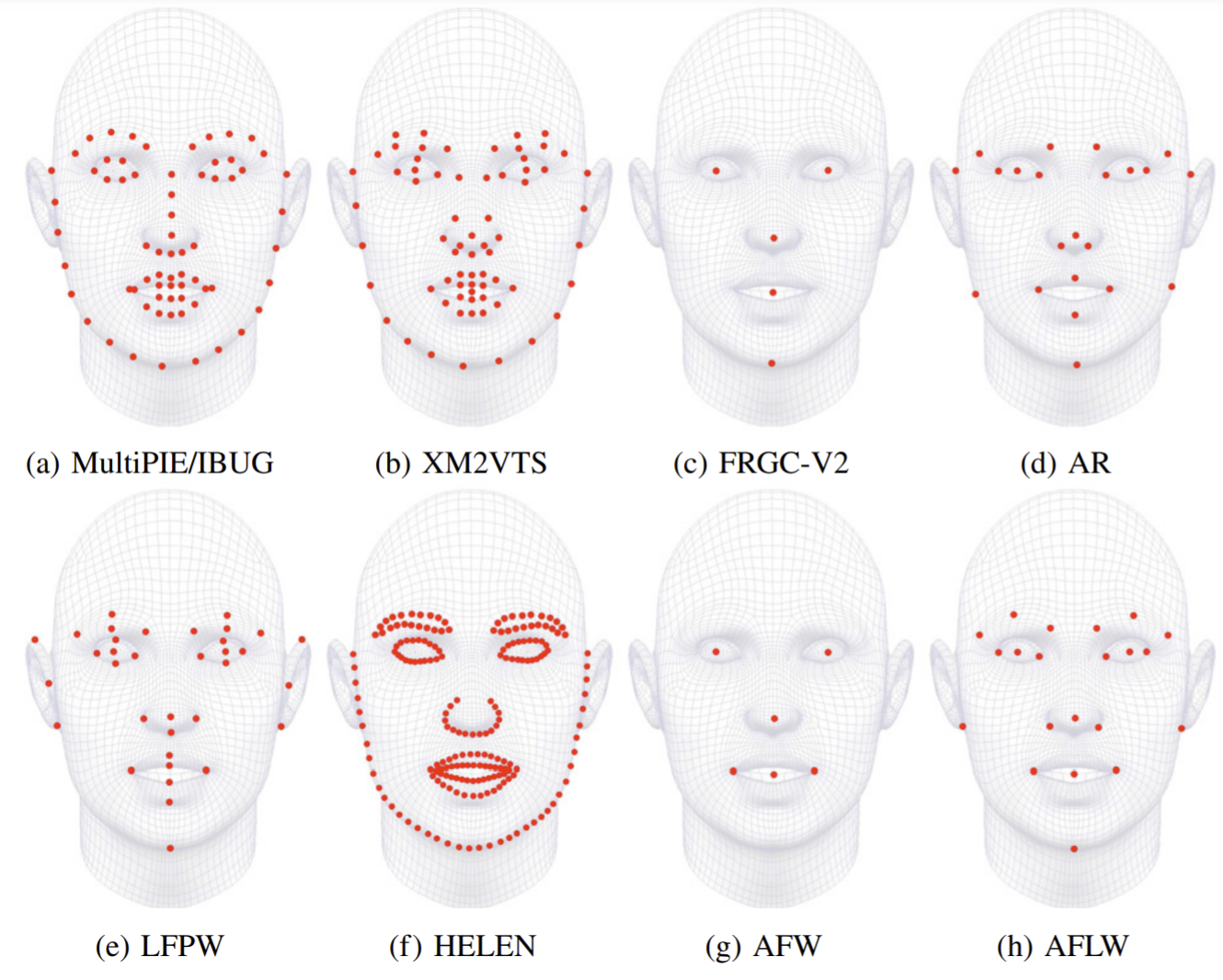
Image Credits: Synthesis AI
Robin Röhm, the cofounder of data analytics platform Apheris, argues that quality checks should be developed for every new synthetic dataset to prevent misuse. The party generating and validating the dataset must have specific knowledge about how the data will be applied, he says, or run the risk of creating an inaccurate — and possibly harmful — system.
Behzadi agrees in principle — but with an eye toward expanding the number of applications that Synthesis supports, beating back rivals like Mostly AI, Rendered.ai, YData, Datagen, and Synthetaic. With over $24 million in financing and Fortune 50 customers in the consumer, metaverse, and robotics spaces, Synthesis plans to launch new products targeting new and existing verticals including photo enhancement, teleconferencing, smart homes, and smart assistants.
“With an unrivaled breadth and depth of representative human data, Synthesis AI has established itself as the go-to provider for production-level synthetic data … The company has delivered over 10 million labeled images to support the most advanced computer vision companies in the world,” Behzadi said. “Synthesis AI has 20 employees and will be scaling to 50 by the end of the year.”